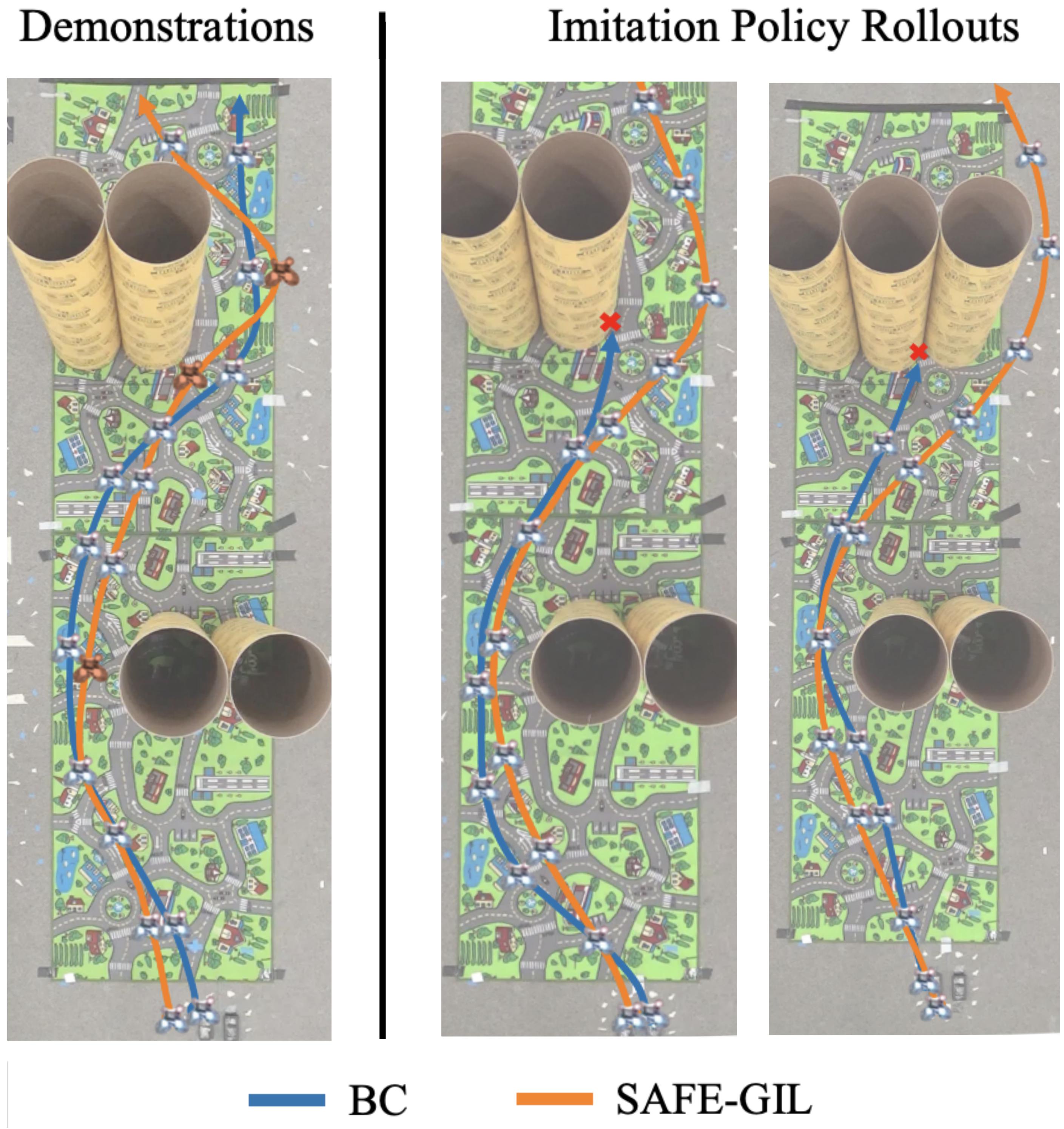
Behavior cloning (BC) is a widely-used approach in imitation learning, where a robot learns a control policy by observing an expert supervisor. However, the learned policy can make errors and might lead to safety violations, which limits their utility in safety-critical robotics applications. While prior works have tried improving a BC policy via additional real or synthetic action labels, adversarial training, or runtime filtering, none of them explicitly focus on reducing the BC policy's safety violations during training time. We propose SAFE-GIL, a design-time method to learn safety-aware behavior cloning policies. SAFE-GIL deliberately injects adversarial disturbance in the system during data collection to guide the expert towards safety-critical states. This disturbance injection simulates potential policy errors that the system might encounter during the test time. By ensuring that training more closely replicates expert behavior in safety-critical states, our approach results in safer policies despite policy errors during the test time. We further develop a reachability-based method to compute this adversarial disturbance. Our method demonstrates a significant reduction in safety failures, particularly in low data regimes where the likelihood of learning errors, and therefore safety violations, is higher.
[Paper] [Project Website]